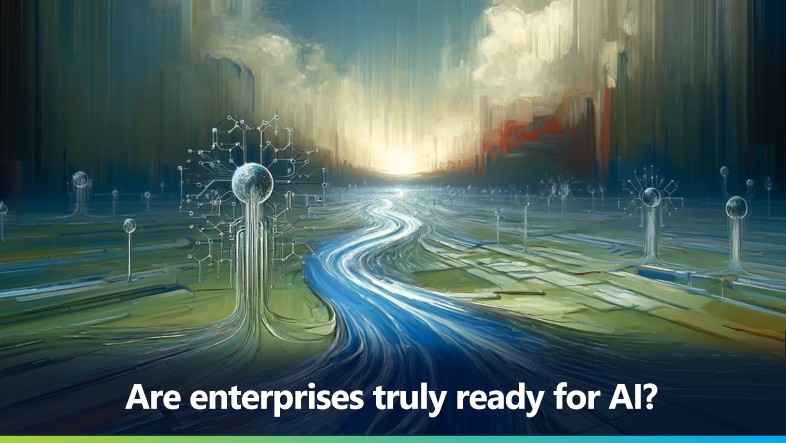
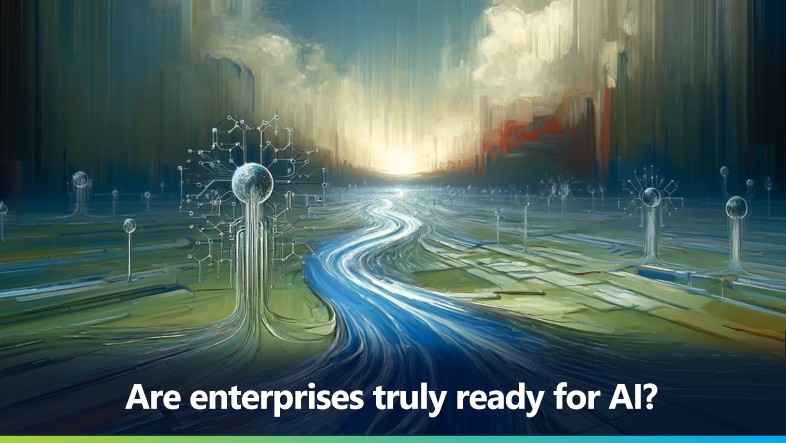
Table of Contents
The potential of Artificial Intelligence (AI) has become undeniable in the world of business. It’s no longer a question of if AI will transform industries, but a matter of how and when. I notice enterprises diving headfirst into AI initiatives. But the truth is, most of these forays remain departmental experiments, lacking the scale and strategic focus to reap the full benefits AI holds. Let’s delve into the roadblocks and outline how we can realize those promised benefits that keep AI at the top of our priority lists.
The Perils of AI in Silos
All too often, I see the excitement around AI manifest in departmental silos. A marketing team might build customer segmentation models, and operations might tackle a supply chain optimization problem. These localized efforts are valuable, proving the concept of AI, but they don’t position the enterprise to be truly transformed by it. Why? Several reasons:
Wasted Resources: Teams reinvent the wheel, duplicating data preparation or model development efforts instead of benefiting from shared infrastructure and knowledge.
Missed Opportunities: AI’s strength lies in seeing patterns that we humans can’t. When data is restricted to just one department’s view, the insights are inherently limited.
Scaling Woes: Solutions built-in isolation rarely translate smoothly across the business; compatibility and integration are afterthoughts.
Stifled Innovation: The lack of cross-department collaboration limits the ‘what if’ scenarios that could spark breakthrough AI use cases.
The Cornerstone of AI Success: A Data-Centric Foundation
The adage “garbage in, garbage out” is amplified with AI. Models are only as insightful as the data they’re fed. I’ve witnessed many enterprises struggle because their data lives in scattered, incompatible systems. CIOs need to shift that conversation. Before talking about fancy neural networks, we must make data pipelines the priority. Here’s what this means:
Holistic Vision: It’s about a centralized, accessible data lake—not just aggregated, but truly integrated and enriched.
Quality Above All Else: Cleansing, standardization, and governance aren’t exciting, but they make or break AI projects.
Real-Time Readiness: The ‘good enough’ batch processes of yesterday won’t cut it when AI needs to inform split-second decisions.
Security & Compliance By design, more data in one place means more risk. Strong safeguards must be baked in, not bolted on later.
What a Robust Data Pipeline Enables
Training with Confidence: AI models devour data. Without a pipeline, the quality and representativeness of that fuel are suspect.
Data-Driven Innovation: We want analysts and business users to explore data for themselves, finding new AI angles. That only works when the data is trustworthy.
The ‘Aha!’ Moments: The magic of AI is revealing what we don’t know to ask. This requires the ability to connect the dots across all of a company’s data.
Beyond the Data: Skills And the Bottom Line
Of course, robust pipelines alone won’t guarantee AI success. Enterprises grapple with securing the necessary talent to make it all work. Finding that delicate balance between in-house expertise and partnerships is crucial. Here is a two-pronged approach:
Upskilling from Within: Employees with deep institutional knowledge can be invaluable AI assets with the right training. Investing in reskilling or targeted AI education programs fosters internal talent loyal to the organization’s goals.
Strategic Outsourcing: When highly specialized skills (think advanced natural language processing or niche computer vision expertise) are needed, partnering with AI consultancies or vendors can bridge the gap. This allows for focused investment without the commitment of a full-time hire.
Addressing Budgets and ROI:
Budget battles are real. That’s where CIOs must champion AI investment, not as a moonshot but as foundational infrastructure—the cost of doing business in the 21st century.
Here’s the crux of the argument that resonates with the C-suite: budget. CIOs need to reframe the AI conversation away from experimental gambles toward essential infrastructure.
The Competitive Edge: Framing AI not as an option but as a necessity for survival in the data-driven economy. Competitors are already leveraging AI; falling behind erodes market share.
Long-Term Savings: Yes, upfront costs are high, but AI done right improves efficiency. Automation, smarter decision-making, and streamlined processes translate into cost savings over time. It’s analogous to upgrading outdated IT infrastructure for long-term gain.
Intangible but Impactful: AI’s potential to unlock innovation is harder to quantify. CIOs can point to case studies in the same industry where AI-enabled new revenue streams or drastic improvements in customer experience. Emphasize the missed opportunity cost of not investing
Risk Mitigation: Failure to adopt AI poses its own risks. Outdated business models become more vulnerable to disruption. AI can be instrumental in identifying threats and proactively addressing them.
The Path to Transformative AI
Building a robust data pipeline is, frankly, hard work. But it’s what separates the AI hype from AI-powered enterprises. By taking ownership of data as CIOs, and fostering a culture of collaboration, CIOs can overcome hurdles. Let’s focus on high-impact AI use cases and clearly showcase the ROI to our business partners. AI won’t transform companies overnight, but with a strategic, data-first approach, its potential is undeniable. CIOs have to play a role in steering enterprises towards truly realizing that potential.