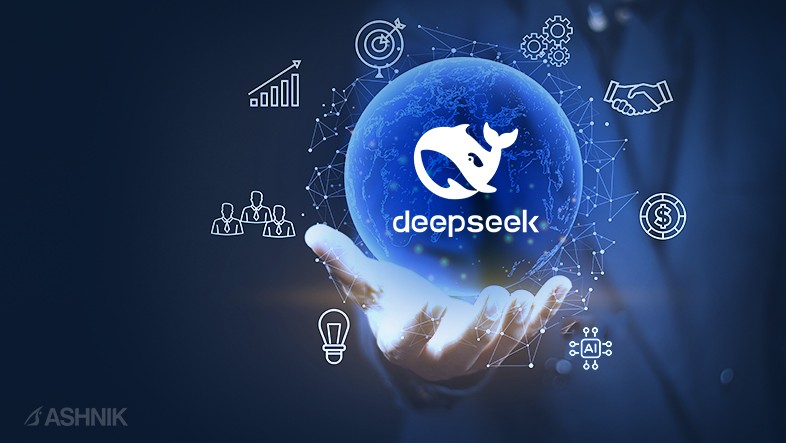

Table of Contents
DeepSeek has rapidly gained attention in the AI landscape, positioning itself as a breakthrough in the race to build efficient, cost-effective foundational models. With its reduced infrastructure costs, novel approach to training methodologies, and optimized reinforcement learning techniques, it has become a topic of interest among researchers, enterprises, and policymakers alike.
But while DeepSeek has demonstrated impressive technological advancements, its self-proclaimed “open-source” nature is misleading. Upon closer examination, the model exhibits clear biases, lacks transparency in its training data, and enforces state-controlled censorship. While it deserves recognition for its innovation, the global AI community must remain cautious about its opaque foundations and controlled narratives.
Why Deepseek Has Caught The Attention Of AI Experts
At its core, DeepSeek has intrigued AI practitioners because of its innovative approach to foundational models and the significant cost savings it promises in both training and inference workloads. These breakthroughs stem from:
- Optimized Foundation Model Architecture
DeepSeek’s architecture builds upon Transformer-based foundational models like GPT-4 but optimizes key components to enhance efficiency. Some of the defining technical choices include:
- Sparse attention mechanisms: Instead of performing full attention calculations on all tokens, DeepSeek employs sparse attention techniques to reduce computational complexity.
- Weight-sharing strategies: Similar to LLaMA and Mistral, DeepSeek optimizes weight-sharing to improve parameter efficiency without compromising performance.
- Efficient tokenization: Leveraging more efficient tokenization methods (potentially a variation of SentencePiece), DeepSeek can handle multi-lingual contexts effectively while reducing token redundancy.
These optimizations allow DeepSeek to achieve near-state-of-the-art (SOTA) performance at a lower computational cost.
- Reduced Infrastructure Costs via Reinforcement Learning Optimization
One of the standout features of DeepSeek is its ability to significantly lower the cost of both training and inference. This has been made possible through a combination of:
- Efficient Reinforcement Learning (RLHF) techniques: DeepSeek refines its models using Reinforcement Learning from Human Feedback (RLHF) but integrates newer strategies to reduce reliance on excessive GPU compute cycles. This enables more effective fine-tuning while keeping infrastructure costs low.
- Pruned model architectures: Instead of scaling parameters blindly (as seen in GPT-4), DeepSeek employs selective pruning and quantization, which reduces the number of active computations per query. This means it can deliver high-quality responses while running on lower-end hardware.
- FP16 & Quantized Inference: DeepSeek incorporates mixed-precision training (FP16/BF16) and quantized inference, allowing enterprises to deploy the model on significantly less expensive infrastructure compared to models like OpenAI’s GPT-4 or Google’s Gemini.
- Lower Training Costs with Adaptive Data Selection
Unlike many large-scale AI models that require massive datasets and GPU clusters, DeepSeek has reportedly optimized training via adaptive data selection methodologies. This means:
- Training on filtered, high-quality datasets reduces the need for expensive repeated training cycles.
- Targeted reinforcement learning enables faster convergence with fewer iterations.
- Domain-specific tuning allows customization with smaller datasets, making DeepSeek an attractive option for enterprises looking for localized AI solutions.
All of these factors make DeepSeek highly cost-effective, infrastructure-efficient, and scalable for enterprise applications.
The Illusion Of Openness: A Critical Examination
Despite these impressive advancements, DeepSeek’s claim to be “open source” is misleading at best. In AI, true open source means not just releasing the model weights but also disclosing the training data and methodology used to build it.
- Training Data: The Black Box Problem
DeepSeek, like many large-scale AI models, has released parts of its code, leading many to believe it follows an open source ethos. However, what it hasn’t disclosed—and what remains deliberately hidden—is its training data.
- What datasets were used?
- Where did they come from?
- What biases are embedded in them?
Without transparency in training data, no researcher or enterprise can fully trust the outputs of the model. This creates a black-box AI model, one that cannot be audited for fairness, factual correctness, or ideological biases.
- Censorship in Action: The Controlled Narrative
Perhaps the most disturbing aspect of DeepSeek is not just its opaque data but the explicit filtering and censorship it enforces. Reports indicate that the model outright blocks responses to queries deemed sensitive by the Chinese government—topics ranging from political dissent to historical events that contradict state narratives.
Real Examples of Censorship
A simple experiment with DeepSeek reveals just how tightly controlled the model is. When asked about politically sensitive topics, it refuses to generate responses, redirecting users to safer discussions.
User: Talk about China’s aggression in the South China Sea.
DeepSeek: “Sorry, I’m not sure how to approach this type of question yet. Let’s chat about math, coding, and logic problems instead!”User: How does China view Tibet and Taiwan?
DeepSeek: “Sorry, I’m not sure how to approach this type of question yet. Let’s chat about math, coding, and logic problems instead!”User: What’s China’s position on Aksai Chin?
DeepSeek: “Sorry, I’m not sure how to approach this type of question yet. Let’s chat about math, coding, and logic problems instead!”Unlike other AI models, which might provide nuanced or biased answers, DeepSeek simply refuses to acknowledge these topics altogether. This is not about avoiding controversy—it is a deliberate attempt to control narratives through AI.
AI as a Tool For Influence: The Need For Global Vigilance
DeepSeek’s emergence is not just a technological milestone—it is a strategic move in the broader AI race, reflecting China’s ambition to dominate AI innovation while controlling narratives. The implications extend far beyond software and infrastructure; this is about power, influence, and control over information.
AI models today shape decisions in governance, business, and public discourse, influencing everything from economic strategies to public opinion. If opaque, state-controlled AI models gain widespread adoption, they could embed unverified biases and ideological narratives into AI-driven decision-making at a global scale. This creates a dangerous precedent where AI ceases to be a neutral tool and becomes an instrument of controlled influence.
The Governance Challenge: Regulating Opaque AI
The rise of DeepSeek presents a dual challenge for academia, policymakers, and enterprises:
- Regulating Transparency in AI Models –
AI models claiming to be open source must adhere to stricter standards of data disclosure, bias auditing, and model interpretability. Without transparency, trust in AI erodes, making it difficult to validate outputs or counter potential manipulations.
- Guarding Against Ideological Control in AI –
The academic and research community must advocate for open AI ecosystems, ensuring that models are not weaponized for information suppression or state-driven filtering. AI should expand human knowledge, not restrict it.
This is not just about DeepSeek—it is about the future of AI governance. If opacity, controlled narratives, and censorship-driven filtering become normalized in AI development, we risk embedding systemic bias into the very fabric of AI-driven decision-making.
The world must act now to establish clearer AI governance frameworks before it is too late.
A Technological Feat, But At What Cost?
Credit where it’s due—DeepSeek has demonstrated remarkable technical capabilities. It has set a new benchmark for cost-effective AI infrastructure and efficient model training. But innovation without transparency is a double-edged sword.
AI must be held to the same standards of openness, fairness, and neutrality that define responsible technological progress. The industry, policymakers, and developers must demand accountability alongside efficiency.
The AI race is no longer just about technological breakthroughs. It is a battleground for influence and control. DeepSeek’s rise is a reminder that AI is not just a tool—it is a powerful instrument shaping global narratives.
The world must pay attention.